Why Data Matters in the AI Revolution
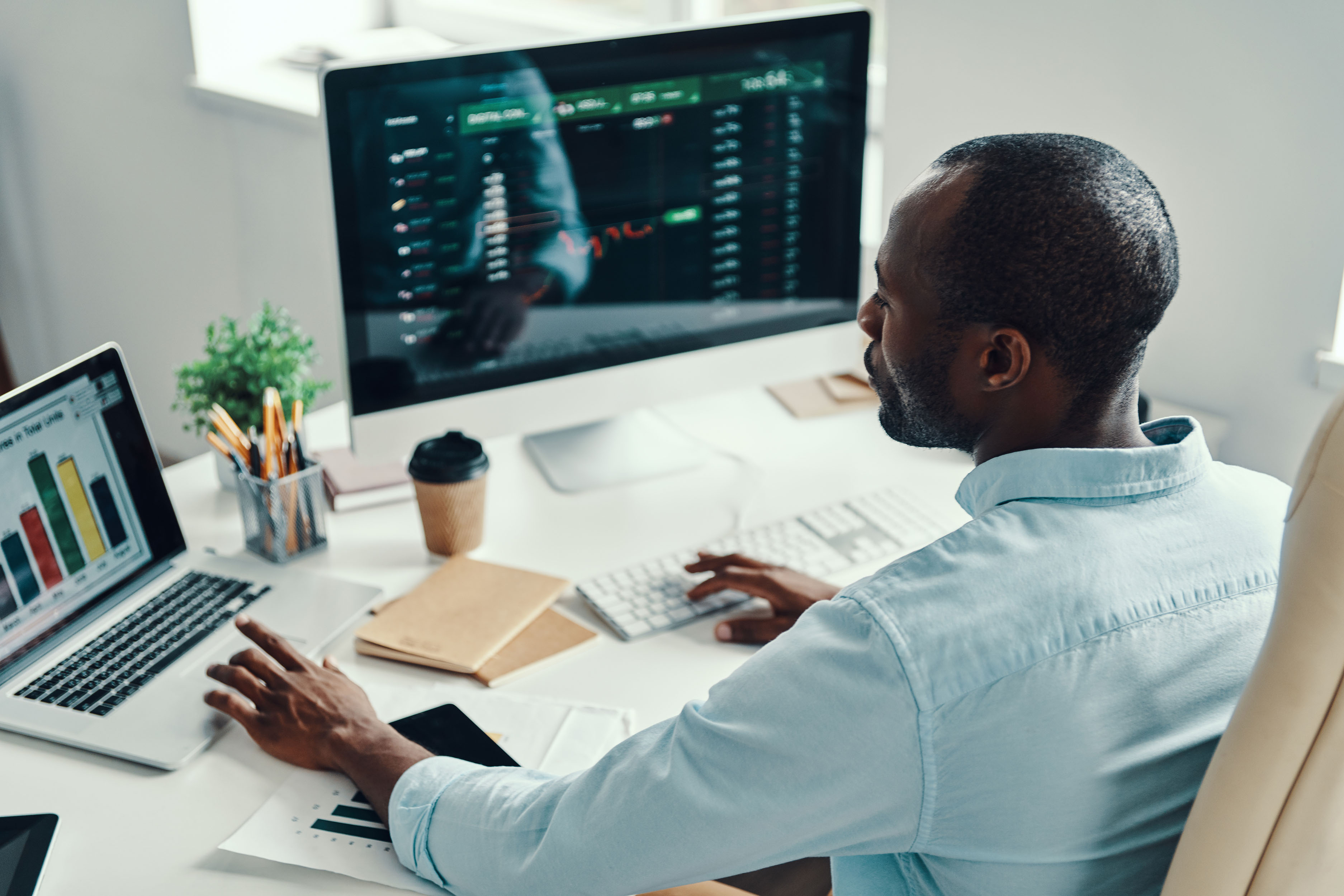
As we witness organizations increasingly relying on AI, it’s clear that the true power of artificial intelligence lies in its ability to analyze vast amounts of data and turn it into actionable insights.
Let's break this down a bit more. AI models are trained on data to recognize patterns, make predictions, and offer solutions. The more high-quality data these models have, the better they become at tasks.
So, what exactly is "data" in the context of AI? Data in AI refers to the vast and diverse sets of information that AI systems use to learn and make decisions. It's not just numbers and spreadsheets; it includes text, images, videos, and even real-time streams of information.
Let's dig deeper to understand what constitutes data in AI, how it's used, and why it's the foundation of the AI revolution.
What is Data in AI?
Data in AI refers to the raw information that is fed into AI algorithms to train and develop models. This data can come from various sources, such as text documents, images, videos, audio recordings, sensor readings, and databases.
The quality and quantity of data significantly impacts the performance and accuracy of AI models. Without data, AI algorithms would have nothing to analyze or learn from, making data the foundational building block for AI technologies.
In the evolution of AI, data has become increasingly important. Early in the history of AI, systems were built on a limited set of rules and static knowledge bases. However, as data collection and storage methods improved, AI systems evolved to utilize massive datasets for more complex tasks.
This shift marked a turning point in the data in AI revolution, where data became the fuel that drives AI innovation. Companies today use data to drive AI applications with the help of AI consulting services to implement these advanced solutions.
Why Data Comes Before AI
Data is the essential fuel that powers AI advancements. Without data, AI algorithms wouldn't be able to function effectively. Here's why data must come first in any AI process:
- Foundation for Training Models: Data provides the foundation for training AI models. To create accurate and useful AI systems, huge amounts of data are needed. The more data you provide, the better the AI learns and performs. In simple terms, data is like a teacher for the AI.
- Understanding Patterns: AI needs data to understand patterns. By analyzing huge sets of data, AI algorithms can find trends, connections, and similarities that humans might miss. This ability to recognize patterns is what allows AI to make predictions and solve complex problems.
- Feedback for Learning: Data gives AI the feedback needed for learning. Just like a student who learns from mistakes, AI improves by comparing its outputs with real-world data, adjusting itself to get better results.
- Customization and Personalization: With enough data, AI can customize and personalize experiences. Whether it’s personalized recommendations on a shopping website or tailored healthcare solutions, AI uses data to understand individual preferences and needs, delivering more relevant results.
- Decision-Making: Data is crucial for decision-making in AI. By analyzing past data, AI algorithms can make informed decisions about future actions, helping businesses optimize operations, predict trends, and improve customer experiences.
Evolution From Early Artificial Intelligence (AI) to Generative AI
The story of artificial intelligence is a fascinating journey of progress and innovation, beginning as a dream of intelligent machines in the 1950s and evolving into the powerful tools we use today.
1950s-1960s: The Birth of AI
This is when the idea of AI was first introduced. John McCarthy, the father of AI, with other scientists and mathematicians, established the field. They started to experiment with simple programs that could perform basic tasks, like playing games or solving math problems. Though these systems were basic, they set the foundation for the AI we know today. The rise of AI began with these early experiments in artificial intelligence.
1970s-1980s: The Rise of Expert Systems
In the 1970s and 1980s, AI development saw the rise of expert systems. These were programs designed to mimic human decision-making by using a set of rules based on specific expertise, such as diagnosing medical conditions or troubleshooting technical issues. These systems were important in industries like healthcare and finance, providing key data insights and AI benefits by automating expert-level tasks.
MYCIN, one of the best examples developed at Stanford University, was an early expert system used to diagnose bacterial infections and recommend antibiotics. It was notable for its ability to explain its reasoning to the users.
1990s: Machine Learning Emergence
By the 1990s, AI had taken a major step forward with the emergence of machine learning. Unlike expert systems that relied on predefined rules, machine learning allowed computers to learn from data and improve over time.
This shift enabled AI systems to become more flexible and capable, laying the groundwork for more advanced AI tools and solutions. Machine learning also marked a turning point in the history of AI development services.
One notable example is the introduction of Support Vector Machines (SVMs) by Vladimir Vapnik and his colleagues. SVMs became a powerful tool for classification and regression tasks and were used in various fields, such as bioinformatics, text categorization, and image recognition.
2000s: Deep Learning Revolution
The 2000s saw the rise of deep learning after Machine Learning, a type of machine learning inspired by the human brain. Deep learning allowed AI to process huge amounts of data and perform complex tasks like image and speech recognition with high accuracy.
This revolution made AI more powerful and widely used in everyday life, offering huge data insights across industries like healthcare, retail, and finance. One of the earliest and most famous applications of deep learning was the recognition of handwritten digits using the MNIST dataset.
2010s: AI in Everyday Applications
In the 2010s, AI became more integrated into our daily lives. From virtual assistants like Siri and Alexa to recommendation systems on Netflix and Amazon, AI was everywhere. Businesses and individuals benefited from AI's ability to process and analyze data quickly, providing smarter solutions and enhancing productivity.
The rise of AI in everyday applications marked a new era of convenience and efficiency. Businesses began to rely heavily on AI development services or companies to gain data insights, improve customer experiences, and optimize operations. AI was no longer just a tool for researchers; it had become a part of mainstream technology.
2020s: Generative AI (Gen-AI) Emerges
In the 2020s, we saw the emergence of Generative AI, also known as Gen-AI. This new form of AI can create content, such as images, text, music, and even video, with minimal human input.
Examples include language models like GPT (Generative Pre-trained Transformer), which can generate human-like text, and tools like DALL·E, which can create images from textual descriptions. According to IBM, 38% of IT professionals at enterprises report their company is actively implementing generative AI.
Generative AI has opened new doors for creative industries and transformed how businesses approach content creation and automation. The benefits of AI have expanded to new areas, offering unprecedented opportunities for innovation.
How Data Plays a Foundational Role in Driving AI Advancements
Data is crucial in advancing artificial intelligence (AI). It serves as the foundation for developing smarter, more effective AI systems. Here’s a look at how data influences AI and its various applications:
- Training AI Models: Data is essential for training AI models. These models learn from vast amounts of data to recognize patterns, make predictions, and perform tasks. For instance, a software development company might use historical sales data to train an AI model that predicts future sales trends. This helps organizations make informed decisions about inventory and marketing strategies.
- Improving Accuracy and Performance: Accurate and high-quality data ensures that AI models perform well. The better the data, the more precise the AI’s predictions and actions. For instance, in IT operations, AI tools for monitoring system performance and predicting failures need clean and accurate data to provide reliable insights. We at In Time Tec provide comprehensive solutions to improve your data quality in AI, ensuring your AI systems operate with maximum efficiency.
- Enabling Personalization: Data allows AI systems to offer personalized experiences. By analyzing user behavior and preferences, AI can customize recommendations and content. For example, cloud service providers use data on user behavior and usage patterns to offer customized recommendations for cloud resource management. To achieve this, companies often hire top AI developers who are experienced in creating algorithms that leverage consumer data to build unique user experiences.
- Powering Advanced Algorithms: Advanced algorithms rely on large datasets to function effectively. For example, in finance, AI algorithms analyze market data to detect fraud patterns or manage investment risks. This helps financial institutions secure transactions and optimize investment strategies.
- Data-Driven Decision Making: Organizations use data-driven insights to make informed decisions, improving strategic planning and operational efficiency. For instance, e-commerce platforms analyze customer data to determine which products to promote, ultimately boosting sales and customer engagement.
- Data as the Basis for Generalization: AI models need to generalize from specific data to handle various situations. High-quality data allows models to learn broader patterns that apply to different scenarios. Organizations that invest in data quality often find that their models are more robust and adaptable, which is key to scaling AI solutions.
Need AI that adapts to various scenarios? We at In Time Tec offer AI development services that ensure your models are trained with diverse data to handle different use cases. - Supporting Explainable AI and Transparency: Transparency in AI is essential for trust and accountability. Data enables AI systems to provide explanations for their decisions. For example, a credit scoring AI can use data to explain why a loan application was approved or denied, helping users understand the decision-making process.
- Enabling Transfer Learning: Transfer learning allows AI models to apply knowledge gained from one task to another related task. Data from previous tasks helps the AI adapt to new challenges more effectively. For example, an AI model trained in image recognition for one type of object can be adapted to recognize similar objects with less additional data.
- Facilitating Innovation in Emerging AI Fields: Data empowers innovation in new AI technologies. By exploring new data sources and types, organizations can develop groundbreaking AI applications. For example, hiring AI developers with expertise in cutting-edge AI fields often focuses on data strategies that enhance these emerging technologies.
So, whether it’s ensuring data quality in AI, improving algorithms, or driving personalization, businesses must prioritize their data strategies. To remain competitive and capitalize on AI’s full potential, it’s essential to hire an AI developer that understands the importance of data and can deliver AI solutions to your requirements.
Conclusion
As we look ahead, the importance of data will only grow. Upcoming trends in AI, such as the rise of autonomous systems, explainable AI, and ethical AI, will all rely heavily on high-quality data. By investing in data governance, quality assurance, and AI development, companies can ensure that their AI systems are equipped to meet the challenges and opportunities of the data-driven era.
Looking for expert guidance on AI and data? Contact In Time Tec for customized solutions and support.
Feel like you could use a hand?
See what’s possible and give your teams the ability to create positive change.
Contact NowElevate your tech savvy! Warning: May cause increased knowledge.
Exclusive technology and development insights, tips, and podcasts await.